Sensing Light
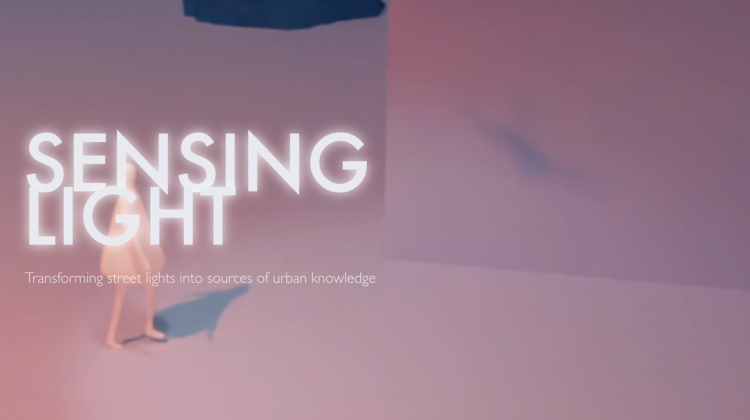
In Sensing Light, we review existing cases of cities that are digitizing their public lighting infrastructure and analyze their various approaches to smart lighting to propose a framework by which we can maximize their potential uses.
We use digital sensors to explore new functional possibilities for street lights, and perform a series of urban demonstrations that leverage air quality monitoring, hyperspectral digital images, and artificial intelligence in order to monitor the utilization of urban spaces, such as curbside space, currently utilized for parking in cities, sidewalks and streets as an example of how to develop interoperability between different urban infrastructure systems.
Finally, we investigate the policy dimensions of transforming a traditional urban infrastructure into a digital urban platform open to the community for development and research.